Professor Mahzarin Banaji is a Professor of Psychology at Harvard University, studying implicit beliefs, values, and social groups. Best known for her pioneering research on implicit bias, Banaji’s insights on the topic of AI offered depth to our interview series. In this conversation, she discusses how a digital archive of language can help us track attitudes across time periods, and how we can use algorithmic data to reflect on our own beliefs. Additionally, she offers a word of caution for algorithm developers and AI innovators: exercise your conscience while creating these new tools.
This interview has been edited for length and clarity:
Could you give us an overview of your research?
Our minds work [in ways] that are unknown to us. This has fascinated me since I was in graduate school. If you and I know different things, I understand that you know ‘A’ and I know ‘B’ and the two are not the same, but what I'm interested in is how a person’s mind can hold two different views on things, or may show an attitude in a positive direction on one kind of measure but show a negative attitude on a different measure,
One way to think about this is to go back in history a little bit and to think about a very famous book from the 1930s called An American Dilemma. A Swedish sociologist by the name of Gunnar Myrdal was invited by the Carnegie Corporation to study what they called the ‘race problem.’ They thought having a Swedish sociologist would make them neutral. When he came, he expected to see rampant racism in the American South. And he did see that. When he went from dinners to factories, to homes to interview people, he found that indeed, there were statements made that demonstrated that the views [of white Americans towards Black Americans] were quite negative. But what he found intriguing was that these people seemed concerned--they were worried. They were not happy. Because, they would say, ‘Look, one of our biggest principles in America is to be egalitarian, to believe that all people should be free. And yet our history is in opposition to that value.’
When Myrdal wrote the book, he didn't call it, ‘the race problem in America.’ He called it An American Dilemma, and the dilemma was that people in the 1930s and ’40s were dealing with a disparity between their values of egalitarianism and freedom on the one hand, and the actuality of our history on the other.
I think what I study, in some ways, is the new American dilemma. When we ask Americans today how they feel about people from two different groups receiving the same opportunities or access to the same resources, pretty much everybody believes that you should be judged by the content of your character, by your talents, and by the work you've done; not by the social groups that you belong to, and not based on whether you were born into a rich family or a poor family. We genuinely, truly believe that. And yet, when we look at the data, what you find, over and over again, are huge disparities. Whether they are gender-based, or race-based, or language, nationality, religion, etc. The problem we confront is that if I'm asked, ‘Do you have any biases? Would you treat two people equally?’ I would say, ‘Of course I would. Why are you bothering me with this silly question?’ But it turns out, if you watch my behavior as a doctor, or as a teacher, or as a judge, or a lawyer, or any person doing any ordinary job, you will see that those very people who profess to be true egalitarians aren't behaving that way. For example, the data show that Black Americans get prescribed lower amounts of painkillers for reporting the same level of pain. The data show that two students who misbehave in the same way, maybe shoving another kid, get treated very differently, depending on gender, depending on race, depending on social class. This is the new American dilemma. How is that happening? And, what needs to happen for there to be more alignment between belief and behavior?
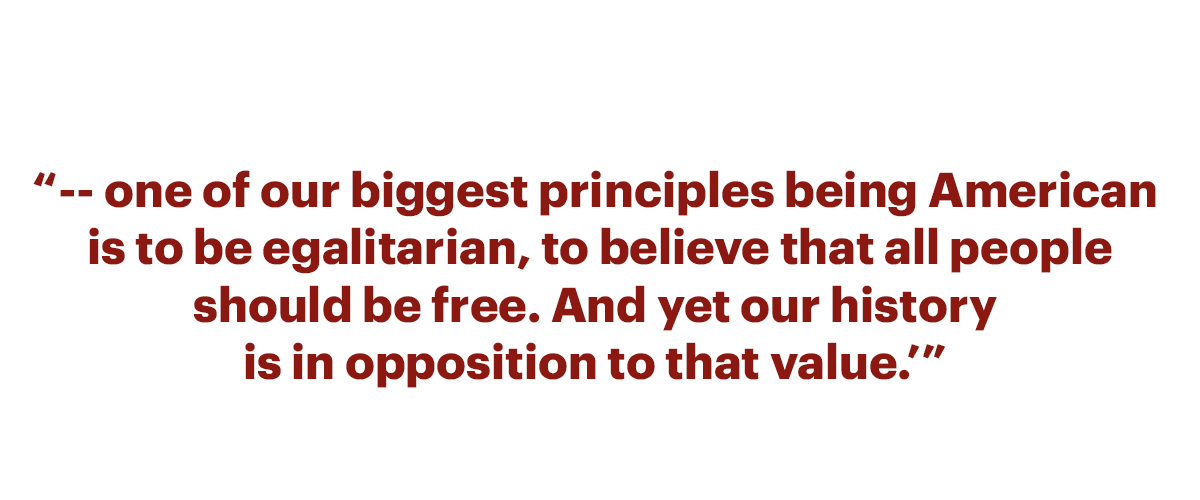
What is important about this work? Why is it timely?
If we know the data on implicit bias, not explicit bias, what we're going to see is that by understanding it, by recognizing it, and by looking at the evidence, rather than our intuition, we will develop a greater capacity to be more accurate in our decision making.
Imagine you have two candidates before you, but you only hire one. What if I collect data that shows the person you did not hire was the better person? What if we can show you that by knowing about implicit bias, you will be more likely to make better decisions? So, that is point number one. And the second is, I think, an even more persuasive reason. What if I told you that by understanding implicit bias, you will be able to bring your own behavior more in line with your own values?
Whatever my values are, I'm totally committed to making sure that I behave in line with [them]. The last 50 to 60 years of my field, experimental psychology, has consistently shown that is not the case. People intend well, and they speak well. Their values read like the constitutions of countries. But, when you look at the nitty-gritty behavior, it's not in line. And the reason is that it's not consciously accessible to us. People are not bad people. They're not acting out of malice. They don't hate any group. They're unaware and they can't be aware until technology is developed to reveal to them what their minds are actually doing.
My students and I have been at work for the last four decades, or so, doing research to develop methods that will allow us to reveal to ourselves what might have dropped into our heads that we don't even know exists. What is that thumbprint of the culture on my brain? Reveal it to me.
Why is this kind of insight important for businesses?
People in the business world need to understand that they should think about the word bias not as a good or a bad thing, but rather, as neutrality and deviation from neutrality. The business world has dealt with deriving data largely by surveys, by asking people, ‘How much do you like working here?’ ‘How long will you stay here?’ ‘Are you happy with your manager?’ I'm skeptical of that type of data. I believe that in the business world, there is a place to start introducing these more indirect or implicit measures of our group identity, of our stereotypes, [and] of our attitudes.
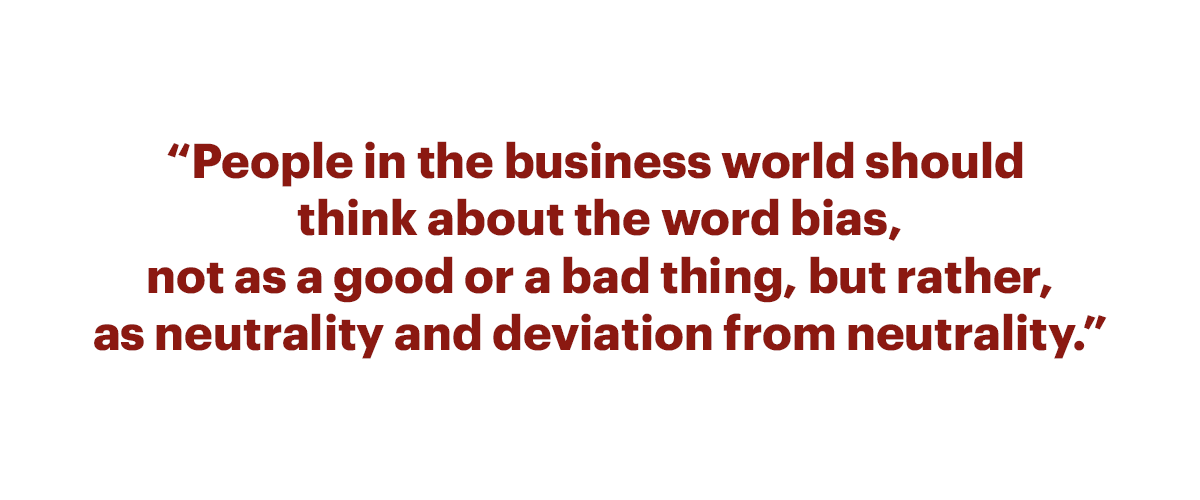
Let's transition a little bit to the theme of today: AI.
We know that the introduction of algorithms in certain decision-making can actually be very good. Places that do health research show us that an algorithm can detect a tumor, or another malady, X percentage times better than the average radiologist. That’s very good. If an algorithm is catching more real tumors that a human was missing, that's what we want AI for, and it is already doing that in some cases.
But there are many other instances in which AI has been introduced without testing and where we should have reason to worry and worry quite deeply. There are algorithms that look at facial tics and movements during a job interview, and feed that data to the hiring manager. Somehow, it will be interpreted to say that ‘this’ kind of twitching is not as good as ‘that’ kind of facial twitching. Yet we have no evidence about the validity of what is being measured.
Then, of course, there's the problem that algorithms are not transparent. Even for the people who wrote those algorithms, the complexity of what is coming out is so great that they themselves cannot unpack it. I think even the builders of that would [not] be able to tell you what it’s actually doing under the hood.
In another example, a judge must decide if a person is likely to repeat their crime. In one method, a model gets fed information about the person: where they live, where they grew up, what they've done in their past, etc. The algorithm then reports to the judge its recommendation for the sentence or the cost of bail. These are places where we should worry a great deal. We should worry because it diminishes the responsibility that judges have traditionally held, replacing it with evidence that's coming from an algorithm we may not fully understand. How fair is it? How transparent is it? How accountable is it? We put these questions together in four letters we’re calling F.E.A.T.: fairness, equity, accountability, and transparency.
What I talked about in the symposium today is work that I've been doing with a computer scientist and a psychologist. We now have access, really for the first time, to very large corpora of language that we are analyzing. One of the results shows that if we look at all of these different groups—gender, unique American ethnic groups, as well as religious groups—there are certain stigmas. For example, if a person is physically disabled and in a wheelchair, one should not assume that their mental capacity is limited. But when we look at one of our tests, we'll see that we incorrectly believe that people who are physically disabled are also mentally disabled. That's a belief that our mind has. If I'm working in a domain where disability is an issue, I ought to know that.
What we believe about groups has been changing over the 200 or so years, but one of the things that we find in the language corpora is that over time, even though our beliefs about who we think different groups are changed, something that underlies these words, their affect or their emotion, whether a negative or positive connotation, has remained pretty much stable over those 200 years.
You can go into 850 billion words that make up something called the “common crawl,” which is basically two snapshots of the internet taken in 2014 and 2017 over a two-week period. We can go into that dataset and say, “What do people think about the categories male and female?” We're finding that our language is drenched with stereotypes. Our language is drenched with attitudes that we would not think we should even have.
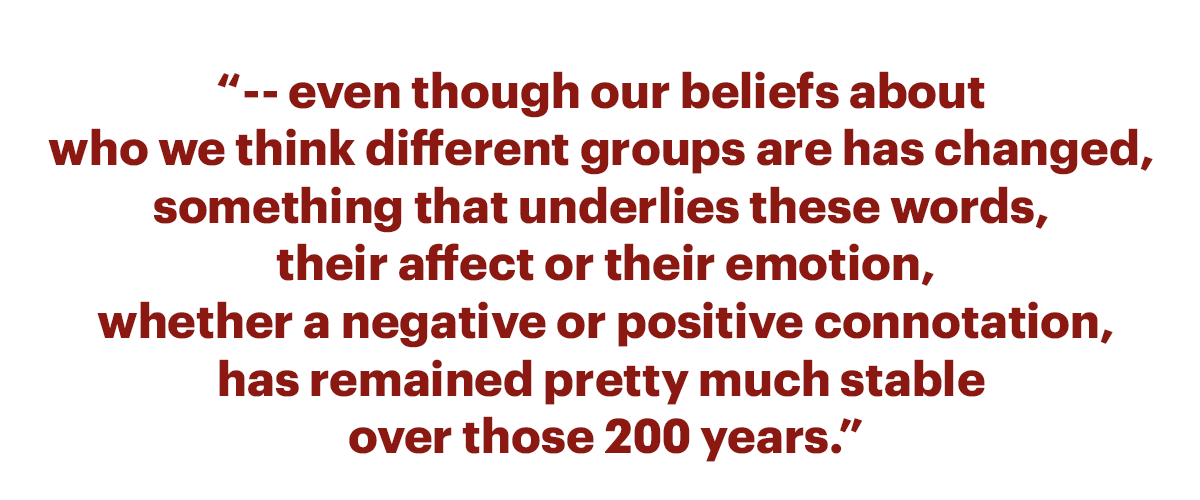
How do you see the future research on AI as it pertains to inequality?
I think that we are already on a very good path. I will say, you know, sometimes fields produce something that could be dangerous—take physicists. They made something called an atom bomb. It was necessary and needed given the period, but as everybody who's watched Oppenheimer knows, the building of the bomb led to so many questions. “Who are we to play God? How could we do this?”
What are we going to do to protect ourselves? While everybody is going to use AI, the people who actually build it come from a few different disciplines, but largely computer science. What's encouraging to me is that the community is incredibly mindful of the problems of AI. There are large numbers of people studying bias in AI. Institutes are cropping up whose main job is to focus on these issues. I mentioned to you the algorithmic justice group at the Santa Fe Institute, many of whose members have been involved in working with the New Mexico state government. I think we will be okay, but only if we don't let corporate interests drive how this work unfolds.